Regional Corn Modeling at a High Resolution Scale: A Yield Based Approach and Blue vs Green Water Assessment
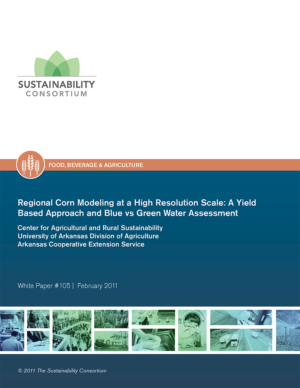
Author(s): | [doc_author] |
Date Published: | [doc_create_date] |
Projects: | [projects] |
Number of Downloads | 20193 |
Size | 1.9 MB |
File | Action |
---|---|
Regional Corn Modeling at a High Resolution Scale (PDF) | Download |
Global water resource demand is expected to increase by as much as 50 percent in the next 40 years as the human population reaches nine billion. Agricultural water use currently accounts for as much as 70 percent of total water use. Increasing demand for food, feed, fiber and fuel will increase demand for irrigation water and put greater pressure on an already stressed resource. In addition, global climate change will shift rainfall patterns, compounding water stress in certain regions. Predicting future cropping patterns is essential to ensure a sustainable world for a growing human population.
The goal of this project was to develop a crop water demand simulation process incorporating the CERES-Maize model in the Decisions Support System for Agrotechnology Transfer (DSSAT) Cropping System Model (CSM) program, version 4.0 (Jones & Kiniry, 1986; Jones, et al., 2003; Hoogenboom, et al., 2004). The CERES-Maize model, part of DSSAT, was chosen for the study as it represents one of the most tested and widely used crop simulation models available. The model simulates six corn phenological stages and the resulting biomass production, and grain yield using site specific input data. Final yield is determined by soil, weather, and management characteristics such as fertilizer application, irrigation, planting and harvesting date, plant poplulation, and others. The goal of this project was to simulate global corn yield based on a range of input parameters at the highest geospatial resolution supported by the data. A customized user-interface in the MATrix LABoratory program (MATLAB) was created to allow for the input of global datasets within DSSAT.